A feed-forward backpropagation neural network method for remaining useful life pbkp_rediction of Francis turbines
Abstract:
In this paper, a method based on feed-forward backpropagation artificial neural networks is developed to achieve a more accurate pbkp_rediction of the useful life of the Francis turbines, subject to the monitoring of the condition. Pbkp_redicting the remaining life of the Francis turbine components is critical to an effective condition-based maintenance to improve reliability and reduce overall maintenance costs. With the correct instrumentation it is possible to periodically measure and calculate the necessary operating parameters and, in the present investigation, having input data, the vibration severity in speed magnitude and the turbine efficiency, there will be trained a feed-forward backpropagation neural network in such a way as to obtain the Weibull failure rate function of the Francis Turbine. A two-element input vector is introduced with 100 samples for each input; the targets (100 samples) of Weibull failure rate function are also introduced. The method developed, for its consistency and effectiveness, can be generalized to systems and rotating equipment.
Año de publicación:
2017
Keywords:
- Francis turbine
- neural network
- Weibull distribution
- Remaining life
Fuente:
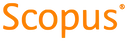
Tipo de documento:
Conference Object
Estado:
Acceso abierto
Áreas de conocimiento:
- Ciencias de la computación
- Red neuronal artificial
- Ciencias de la computación
Áreas temáticas:
- Métodos informáticos especiales