A fuzzy cognitive map (FCM) as a learning model for early prognosis of seasonal related virus diseases in tropical regions
Abstract:
Fuzzy Cognitive Maps (FCMs) and current developments in Machine Learning have been contributing in capturing human behaviors through data and learning models, which focus on pbkp_redicting, interpreting or identifying behavioral patterns on systems and their relationships. In recent years seasonal diseases caused by vectors that transport viral pathogens in tropical regions, such as the Aedes Aegypti mosquito, have caused noticeable impacts both on public health and country's economies in Latin America. This work proposes a model for early prognosis based on FCMs for making a risk assessment of potential presence of seasonal virus-related diseases in a specific region of the Ecuadorian coast. The FCM is used as a knowledge representation strategy for the cause-effect relationships; and, learning models for gaining the identification of the underlying cause of symptoms. The model aims to improve the chances of proper prognosis of seasonal diseases, which could impact not only the prescription and correct decisions, but also the actions taken for preventing the spread of seasonal diseases.
Año de publicación:
2019
Keywords:
- Tropical Seasonal Diseases
- Machine learning
- Causal Complex Systems
- Dengue fever
- Knowledge Representation
- Fuzzy Cognitive Maps
Fuente:
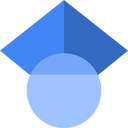
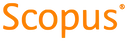
Tipo de documento:
Conference Object
Estado:
Acceso restringido
Áreas de conocimiento:
- Epidemiología
- Inteligencia artificial
Áreas temáticas:
- Salud y seguridad personal