A hierarchical clustering method: Applications to educational data
Abstract:
The use of graphical probabilistic models in the field of education has been considered for this research. First, classical learning algorithms, as PC or K2 are reviewed. But the problem with these general learning procedures comes from the presence of a high number of variables that measure different aspects of the same concept, as it can be the case of socio-economic indicators in a population. In this case, we have that all the variables have some degree of dependence among them, without a true causal structure. So, a new procedure is presented which makes a hierarchical clustering of the data while learning a joint probability distribution. It generalizes AutoClass EM clustering allowing more complex models. Hierarchical clustering is compared in the experiments with classical learning algorithms showing a similar performance when considering the estimation of a joint probability distribution for all the variables, but with a clear advantage: the simplicity and easiness of the interpretation of the model. The method is applied to the analysis of two datasets of the educational data: socio-economic, academic achievement and drop outs at the Engineering Faculty of Quevedo State Technical University, and student evaluation of teachers from Gazi University in Ankara (Turkey).
Año de publicación:
2016
Keywords:
- hierarchical clustering
- ACADEMIC PERFORMANCE
- Bayesian networks
- student evaluation
- EM
- K2
- Pc
Fuente:
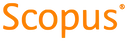
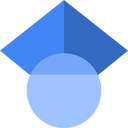
Tipo de documento:
Article
Estado:
Acceso restringido
Áreas de conocimiento:
- Pedagogía
- Tecnología educativa
Áreas temáticas:
- Funcionamiento de bibliotecas y archivos