Applications of Deep Learning in Financial Intermediation: A Systematic Literature Review
Abstract:
In finance, an infinite amount of datais generated daily, which is important for decision-making in the business world. Consequently, there is a need to create models that help to process and interpret this data. Deep learning has demonstrated important advances in the processing of large amounts of data, and for this reason, the objective of this systematic review of literature corresponds to the search for applications, deep learning model and techniques that were used to solve problems in the financial area. For this purpose, out of 346 articles found, 20 were selected that met the inclusion and exclusion criteria corresponding to the research questions. Among the most common applications, models, and techniques were: prediction in market actions, sales forecasting, detection of fraud risks and tax evasion; with respect to the models, convolutional neural networks CNN and recurrent neural networks RNN were among the most executed; the ReLu and Sigmoid techniques turned out to be the most used in these models.
Año de publicación:
2018
Keywords:
Fuente:
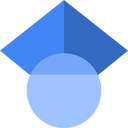
Tipo de documento:
Other
Estado:
Acceso abierto
Áreas de conocimiento:
- Finanzas
- Aprendizaje automático
- Finanzas
Áreas temáticas de Dewey:
- Programación informática, programas, datos, seguridad
- Economía financiera
- Métodos informáticos especiales