Applied LSTM neural network time series to forecast household energy consumption
Abstract:
In Ecuador, energy consumption is accentuated in the residential sector due to population growth and other parameters, which leads to an increase in energy costs, greenhouse gas emissions and fossil fuel subsidies. Hence, there is a need to optimize and reduce energy consumption in buildings. One approach considered is predictive control systems, for which high accuracy consumption predictions are required. In this work we will apply supervised machine learning techniques using neural networks to forecast the energy consumption behavior of a family house; for this purpose, an experimental design is proposed using a dataset of almost four years of energy measurements, four different Long Short-Term Memory (LSTM) architectures are tested and about 200 models are run by varying hyperparameters. Metrics such as root mean square error (RMSE), mean absolute error (MAE) and mean absolute percent error (MAPE) are considered to compare and select the best LSTM model, being the best simple LSTM structure with vectorial output.
Año de publicación:
2021
Keywords:
- Energy efficiency
- TIME SERIES
- forecasting
- LSTM
- buildings
Fuente:
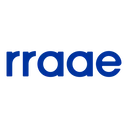
Tipo de documento:
Article
Estado:
Acceso abierto
Áreas de conocimiento:
- Energía
- Energía
- Energía
Áreas temáticas de Dewey:
- Métodos informáticos especiales
- Religión
- Física aplicada

Objetivos de Desarrollo Sostenible:
- ODS 7: Energía asequible y no contaminante
- ODS 13: Acción por el clima
- ODS 9: Industria, innovación e infraestructura
