A machine learning approach for traffic-noise annoyance assessment
Abstract:
In this study, models for predicting traffic-noise annoyance based on noise perception, noise exposure levels, and demographics were developed. By applying machine-learning techniques, in particular artificial neural networks (ANN), support vector machines (SVM) and multiple linear regressions (MLR), the traffic-noise annoyance models were obtained, and the error rates compared. A traffic noise map and the estimation of noise exposure for the case study area were developed. Although, it is quite evident that subjective noise perception and predicted noise exposure levels strongly influence traffic-noise annoyance, traditional statistical models fail to produce accurate predictions. Therefore, a machine-learning approach was applied, which showed a better performance in terms of error rates and the coefficient of determination (R2). The best results for predicting traffic-noise annoyance were obtained with the ANN model, obtaining 42% and 35% error reduction in training subsets compared to the MRL and SVM models, respectively. For testing subsets, the error reductions were 24% and 19% for the corresponding models. The coefficient of determination R2 increased 3.8 and 2.3 times using ANN compared to MRL and SVM models in training subsets respectively, and 1.7 times (in both MRL and SVM models) for testing subsets. In this way, the applied methodology can be used as a reliable and more accurate tool for determining the impact of transportation noise in urban context, promoting the well-being of the population and the creation of suitable public policy.
Año de publicación:
2019
Keywords:
- Support Vector Machine
- artificial neural networks
- MACHINE-LEARNING
- Traffic noise
- Noise annoyance
Fuente:
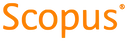
Tipo de documento:
Article
Estado:
Acceso restringido
Áreas de conocimiento:
- Aprendizaje automático
Áreas temáticas de Dewey:
- Física aplicada
- Ingeniería y operaciones afines
- Otras ramas de la ingeniería

Objetivos de Desarrollo Sostenible:
- ODS 3: Salud y bienestar
- ODS 11: Ciudades y comunidades sostenibles
- ODS 9: Industria, innovación e infraestructura
