Artificial Neural Networks for Bottled Water Demand Forecasting: A Small Business Case Study
Abstract:
This paper shows a neural networks-based demand forecasting model designed for a small manufacturer of bottled water in Ecuador, which currently doesn’t have adequate demand forecast methodologies, causing problems of customer orders non-compliance, inventory excess and economic losses. However, by working with accurate pbkp_redictions, the manufacturer will have an anticipated vision of future needs in order to satisfy the demand for manufactured products, in other words, to guarantee on time and reasonable use of the resources. To solve the problems that this small manufacturer has to face a historic demand data acquisition process was done through the last 36 months costumer order records. In the construction of the historical time series, that was analyzed, demand dates and volumes were established as input variables. Then the design of forecast models was done, based on classical methods and multi-layer neural networks, which were evaluated by means of quantitative error indicators. The application of these methods was done through the R programming language. After this, a stage of training and improvement of the network is included, it was evaluated against the results of the classic forecasting methods, and the next 12 months were pbkp_redicted by means of the best obtained model. Finally, the feasibility of the use of neural networks in the forecast of demand for purified water bottles, is demonstrated.
Año de publicación:
2019
Keywords:
- Small business
- Long-term demand forecasting
- artificial neural networks
Fuente:
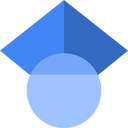
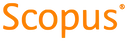
Tipo de documento:
Conference Object
Estado:
Acceso restringido
Áreas de conocimiento:
- Toma de decisiones
- Aprendizaje automático
- Ciencias de la computación
Áreas temáticas:
- Ciencias de la computación