Artificial Neural Networks in Mathematical Mini-Games for Automatic Students’ Learning Styles Identification: A First Approach
Abstract:
The lack of customized education results in low performance in different subjects as mathematics. Recognizing and knowing student learning styles will enable educators to create an appropriate learning environment. Questionnaires are traditional methods to identify the learning styles of the students. Nevertheless, they exhibit several limitations such as misunderstanding of the questions and boredom in children. Thus, this work proposes a first automatic approach to detect the learning styles (Activist, Reflector, Theorist, Pragmatist) based on Honey and Mumford theory through the use of Artificial Neural Networks in mathematical Mini-Games. Metrics from the mathematical Mini-Games as score and time were used as input data to then train the Artificial Neural Networks to pbkp_redict the percentages of learning styles. The data gathered in this work was from a pilot study of Ecuadorian students with ages between 9 and 10 years old. The preliminary results show that the average overall difference between the two techniques (Artificial Neural Networks and CHAEA-Junior) is 4.13%. Finally, we conclude that video games can be fun and suitable tools for an accurate pbkp_rediction of learning styles.
Año de publicación:
2019
Keywords:
- artificial neural networks
- video games
- Learning Styles
Fuente:
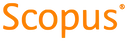
Tipo de documento:
Conference Object
Estado:
Acceso restringido
Áreas de conocimiento:
- Inteligencia artificial
- Tecnología educativa
Áreas temáticas:
- Programación informática, programas, datos, seguridad
- Escuelas y sus actividades; educación especial
- Matemáticas