Artificial intelligence, big data and machine learning approaches in precision medicine & drug discovery
Abstract:
Artificial Intelligence revolutionizes the drug development process that can quickly identify potential biologically active compounds from millions of candidate within a short period. The present review is an overview based on some applications of Machine Learning based tools, such as GOLD, Deep PVP, LIB SVM, etc. and the algorithms involved such as support vector machine (SVM), random forest (RF), decision tree and Artificial Neural Network (ANN), etc. at various stages of drug designing and development. These techniques can be employed in SNP discoveries, drug repurposing, ligand-based drug design (LBDD), Ligand-based Virtual Screening (LBVS) and Struc-ture-based Virtual Screening (SBVS), Lead identification, quantitative structure-activity relationship (QSAR) modeling, and ADMET analysis. It is demonstrated that SVM exhibited better performance in indicating that the classification model will have great applications on human intestinal absorption (HIA) pbkp_redictions. Successful cases have been reported which demonstrate the efficiency of SVM and RF models in identifying JFD00950 as a novel compound targeting against a colon cancer cell line, DLD-1, by inhibition of FEN1 cytotoxic and cleavage activity. Furthermore, a QSAR model was also used to pbkp_redict flavonoid inhibitory effects on AR activity as a potent treatment for diabetes mellitus (DM), using ANN. Hence, in the era of big data, ML approaches have been evolved as a powerful and efficient way to deal with the huge amounts of generated data from modern drug discovery to model small-molecule drugs, gene biomarkers and identifying the novel drug targets for various diseases.
Año de publicación:
2021
Keywords:
- Precision Medicine
- BIG DATA
- Artificial Intelligence
- Drug Discovery
- Virtual Screening
- Machine learning
Fuente:
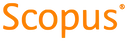
Tipo de documento:
Article
Estado:
Acceso restringido
Áreas de conocimiento:
- Inteligencia artificial
- Ciencias de la computación
Áreas temáticas:
- Ciencias de la computación