A multi-objective approach for anti-osteosarcoma cancer agents discovery through drug repurposing
Abstract:
Osteosarcoma is the most common type of primary malignant bone tumor. Although nowadays 5-year survival rates can reach up to 60–70%, acute complications and late effects of osteosarcoma therapy are two of the limiting factors in treatments. We developed a multi-objective algorithm for the repurposing of new anti-osteosarcoma drugs, based on the modeling of molecules with described activity for HOS, MG63, SAOS2, and U2OS cell lines in the ChEMBL database. Several predictive models were obtained for each cell line and those with accuracy greater than 0.8 were integrated into a desirability function for the final multi-objective model. An exhaustive exploration of model combinations was carried out to obtain the best multi-objective model in virtual screening. For the top 1% of the screened list, the final model showed a BEDROC = 0.562, EF = 27.6, and AUC = 0.653. The repositioning was performed on 2218 molecules described in DrugBank. Within the top-ranked drugs, we found: temsirolimus, paclitaxel, sirolimus, everolimus, and cabazitaxel, which are antineoplastic drugs described in clinical trials for cancer in general. Interestingly, we found several broad-spectrum antibiotics and antiretroviral agents. This powerful model predicts several drugs that should be studied in depth to find new chemotherapy regimens and to propose new strategies for osteosarcoma treatment.
Año de publicación:
2020
Keywords:
- Drug repositioning
- Multi-objective model
- Virtual Screening
- Machine learning
- Osteosarcoma
Fuente:
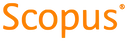
Tipo de documento:
Article
Estado:
Acceso abierto
Áreas de conocimiento:
- Cáncer
- Cáncer
Áreas temáticas de Dewey:
- Fisiología humana
- Enfermedades
- Farmacología y terapéutica