Assessment of Quarterly, Semiannual and Annual Models to Forecast Monthly Rainfall Anomalies: The Case of a Tropical Andean Basin
Abstract:
Rainfall forecasting is essential to manage water resources and make timely decisions to mitigate adverse effects related to unexpected events. Considering that rainfall drivers can change throughout the year, one approach to implementing forecasting models is to generate a model for each period in which the mechanisms are nearly constant, e.g., each season. The chosen pbkp_redictors can be more robust, and the resulting models perform better. However, it has not been assessed whether the approach mentioned above offers better performance in forecasting models from a practical perspective in the tropical Andean region. This study evaluated quarterly, semiannual and annual models for forecasting monthly rainfall anomalies in an Andean basin to show if models implemented for fewer months outperform accuracy; all the models forecast rainfall on a monthly scale. Lagged rainfall and climate indices were used as pbkp_redictors. Support vector regression (SVR) was used to select the most relevant pbkp_redictors and train the models. The results showed a better performance of the annual models mainly due to the greater amount of data that SVR can take advantage of in training. If the training of the annual models had less data, the quarterly models would be the best. In conclusion, the annual models show greater accuracy in the rainfall forecast.
Año de publicación:
2022
Keywords:
- rainfall
- SVR
- SVM
- Anomalies
- Andean river basin
- forecasting
- large-scale climate indices
Fuente:
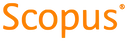
Tipo de documento:
Article
Estado:
Acceso abierto
Áreas de conocimiento:
- Hidrología
- Hidrología
Áreas temáticas:
- Geología, hidrología, meteorología