Augmented Data Deep Learning Model to Pbkp_rediction of S&P500 Index: A Case Study Including Data of COVID-19 Period
Abstract:
The forecasting of the financial markets, as the SP500 Index, is an arduous task because their data is highly noisy, non-linear, complex, dynamic, non-parametric, and chaotic. In this context, this study proposes a two-phase method to pbkp_redict the values of the Index: In the first phase, the Index data are preprocessed and used as input to train a Deep Learning Neural Network (DNN) using augmented data and scaling techniques; in the second phase, the trained model and a sliding window technique are used to pbkp_redict the values in time, step by step. The data set to train and validate the model include the atypical period of COVID-19. According to the results obtained, the model has good performance. Thus, the research offers a new experience in data analysis and can help economic policymakers and institutional investors with accurate forecasts of the stock market.
Año de publicación:
2022
Keywords:
- S&P500
- DNN
- Augmented data
- forecasting
- covid-19
Fuente:
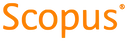
Tipo de documento:
Conference Object
Estado:
Acceso restringido
Áreas de conocimiento:
- Aprendizaje automático
- Finanzas
- Ciencias de la computación
Áreas temáticas:
- Ciencias de la computación