Automated Systems for Detecting Volcano-Seismic Events Using Different Labeling Techniques
Abstract:
Several systems have been developed in the last years to automatically detect volcanic events based on their seismic signals. Many of those systems use supervised machine learning algorithms in order to create the detection models. However, the supervised training of these machine learning techniques requires labeled-signal catalogs (i.e., training, validation and test data-sets) that in many cases are difficult to obtain. In fact, existing labeling schemes can consume a lot of time and resources without guarantying that the final detection model is accurate enough. Moreover, every labeling technique can produce a different set of events, without being defined so far which technique is the best for volcanic-event detection. Hence, this work proves that the labeling scheme used to create training sets definitely impacts the performance of seismic-event detectors. This is demonstrated by comparing two techniques for labeling seismic signals before to train a system for automated detection of volcanic events. The first technique is automatic and computationally efficient, while the second one is a handmade and time-consuming process carried out by expert analysts. Results show that none of the labeling techniques is completely trustworthy. As a matter of fact, our main result reveals that an improved detection accuracy is obtained when machine learning classifiers are trained with the conjunction of diverse labeling techniques.
Año de publicación:
2020
Keywords:
- Machine learning algorithms
- Volcanic seismic events
- Labeled data-sets
- Automated detection systems
Fuente:
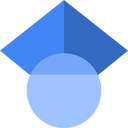
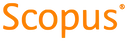
Tipo de documento:
Conference Object
Estado:
Acceso restringido
Áreas de conocimiento:
- Volcanismo
- Análisis de datos
Áreas temáticas:
- Geología, hidrología, meteorología
- Mecánica celeste
- Otros problemas y servicios sociales