Automatic Design of Ensembles of Window Operators for Ocular Image Segmentation
Abstract:
W-operators are a nonlinear class of locally defined and translation invariant image operators. A W-operator is completely defined and represented by a characteristic function, or classifier, that maps a set of window observations to a set of labels. In this work, we propose a new approach to design W-operators for grayscale image processing. In the proposed approach, we constrain both the space of characteristic functions using artificial feed-forward neural networks and the space of observations using the 2-D Haar wavelet transform. The goal of these constraints is to reduce the cost of design and improve the performance of W-operators for practical applications. Based on this approach, a family or set of W-operators is designed and then combined into a single operator using an ensemble method. We evaluated the performance of this approach in the segmentation of blood vessels in ocular images of the DRIVE database. The results show the suitability of this approach: It outperforms W-operators based on logistic regression without any constraint in the space of observations; aperture filters designed using support vector machines and pyramidal multiresolution, and a fast segmentation using the local thresholding method of Otsu.
Año de publicación:
2014
Keywords:
- classification
- wavelet transform
- IMAGE PROCESSING
- segmentation
- Neural networks
- Ensemble of Classifiers
- mathematical morphology
- Ocular Images
Fuente:
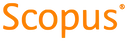
Tipo de documento:
Article
Estado:
Acceso restringido
Áreas de conocimiento:
- Visión por computadora
- Simulación por computadora
Áreas temáticas:
- Métodos informáticos especiales