Automatic Evaluation of Physiotherapy Activities Using Deep Learning Techniques
Abstract:
Physiotherapy activities are necessary in patients with physical disabilities or to restore their functional abilities after injury or surgery. However, home-based therapy requires some mechanisms for automatic evaluation of the correct execution of physiotherapy exercises. Although novel tools and equipment exist to support physical therapy sessions at home, low-cost implementations could fail if patients perform the exercises incorrectly or at an unusual pace. Thus, this work proposes to determine the precision of physical therapy exercises using low-cost motion sensors and deep learning techniques, evaluating the most successful classification architectures based on supervised learning. In particular, the proposed system will classify the type of execution of some common exercises in physiotherapy sessions, labeling them as correct execution, fast execution, or low-amplitude execution. The accuracy and sensitivity results are very promising, as we have obtained an accuracy greater than 95% and an average sensitivity greater than 94% using recurrent neural networks. We hope that works like this will help to improve the effectiveness of physiotherapy sessions at home, leaving aside the need for trained clinicians or restricted schedules.
Año de publicación:
2023
Keywords:
- Exercise evaluation
- Deep learning techniques
- motion sensors
- Physiotherapy activities
Fuente:
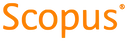
Tipo de documento:
Conference Object
Estado:
Acceso restringido
Áreas de conocimiento:
- Ciencias de la computación
- Aprendizaje automático
- Fisioterapia
Áreas temáticas:
- Farmacología y terapéutica
- Física aplicada