Automatic discrimination between supraventricular and ventricular tachycardia using a multilayer perceptron in implantable cardioverter defibrillators
Abstract:
The morphological analysis of implantable cardioverter defibrillator (ICD) stored electrograms (EGM) using a multilayer perceptron (MLP) has been proposed for discrimination between supraventricular and ventricular arrhythmias. However, a reliable estimation of the accuracy of MLP methods is lacking. The aim of the study was to compare the morphology and spectrum-based MLP with more conventional morphology-based algorithms in a large series of ICD-stored episodes of arrhythmia. One set of ICD-stored electrograms was used for control and training purposes and a second one, consisting of spontaneous episodes in patients with dual chamber ICDs, for validation of the MLP performance. The correlation waveform analysis (CWA) and the EGM width criterion were compared with MLP methods. Bootstrap resampling techniques were used to extract the relevant information in the MLP training. The morphology-based MLP achieved better discrimination than any other method, with areas under the receiver operating characteristic (ROC) curve (tolerance intervals): 0.96 (0.81, 0.96) for MLP, 0.91 (0.77, 0.94) for CWA, and 0.68 (0.49, 0.78) for EGM width in the validation set. A specificity of 73.0% was obtained at 95% sensitivity, compared with 38.1% and 55.1% using CWA and EGM width criteria, respectively. In contrast, the generalization capabilities of spectral-based MLP methods are poor, showing a lower area under the ROC curve in the validation set. Time-domain MLP techniques may be useful for the morphological analysis of the intracardiac EGM signal stored by ICD devices. When properly trained and validated, these methods perform better than other commonly used morphological criteria for discrimination between supraventricular and ventricular arrhythmias.
Año de publicación:
2002
Keywords:
- neural network
- Ventricular tachycardia
- Defibrillator
- electrogram
Fuente:
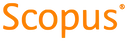
Tipo de documento:
Article
Estado:
Acceso restringido
Áreas de conocimiento:
- Enfermedad cardiovascular
- Aprendizaje automático
- Algoritmo
Áreas temáticas:
- Enfermedades