Automatic feature extraction of time-series applied to fault severity assessment of helical gearbox in stationary and non-stationary speed operation
Abstract:
Signals captured in rotating machines to obtain the status of their components can be considered as a source of massive information. In current methods based on artificial intelligence to fault severity assessment, features are first generated by advanced signal processing techniques. Then feature selection takes place, often requiring human expertise. This approach, besides time-consuming, is highly dependent on the machinery configuration as in general the results obtained for a mechanical system cannot be reused by other systems. Moreover, the information about time events is often lost along the process, preventing the discovery of faulty state patterns in machines operating under time-varying conditions. In this paper a novel method for automatic feature extraction and estimation of fault severity is proposed to overcome the drawbacks of classical techniques. The proposed method employs a Deep Convolutional Neural Network pre-trained by a Stacked Convolutional Autoencoder. The robustness and accuracy of this new method are validated using a dataset with different severity conditions on failure mode in a helical gearbox, working in both constant and variable speed of operation. The results show that the proposed unsupervised feature extraction method is effective for the estimation of fault severity in helical gearbox, and it has a consistently better performance in comparison with other reported feature extraction methods.
Año de publicación:
2017
Keywords:
- deep learning
- Convolution
- Auto-encoder
- Wavelet packets
- Helical gearbox
Fuente:
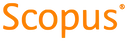
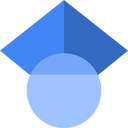
Tipo de documento:
Article
Estado:
Acceso restringido
Áreas de conocimiento:
- Ingeniería mecánica
- Aprendizaje automático
Áreas temáticas de Dewey:
- Física aplicada

Objetivos de Desarrollo Sostenible:
- ODS 9: Industria, innovación e infraestructura
- ODS 12: Producción y consumo responsables
- ODS 8: Trabajo decente y crecimiento económico
