Automatic graph pruning based on kernel alignment for spectral clustering
Abstract:
Detection of data structures in spectral clustering approaches becomes a difficult task when dealing with complex distributions. Moreover, there is a need of a real user prior knowledge about the influence of the free parameters when building the graph. Here, we introduce a graph pruning approach, termed Kernel Alignment based Graph Pruning (KAGP), within a spectral clustering framework that enhances both the local and global data consistencies for a given input similarity. The KAGP allows revealing hidden data structures by finding relevant pair-wise relationships among samples. So, KAGP estimates the loss of information during the pruning process in terms of a kernel alignment-based cost function. Besides, we encode the sample similarities using a compactly supported kernel function that allows obtaining a sparse data representation to support spectral clustering techniques. Attained results shows that KAGP enhances the clustering performance in most of the cases. In addition, KAGP avoids the need for a comprehensive user knowledge regarding the influence of its free parameters.
Año de publicación:
2016
Keywords:
- Kernel alignment
- Graph pruning
- Spectral clustering
Fuente:
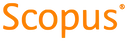
Tipo de documento:
Article
Estado:
Acceso restringido
Áreas de conocimiento:
- Aprendizaje automático
- Algoritmo
- Ciencias de la computación
Áreas temáticas de Dewey:
- Métodos informáticos especiales

Objetivos de Desarrollo Sostenible:
