Automatic motion segmentation via a cumulative kernel representation and spectral clustering
Abstract:
Dynamic or time-varying data analysis is of great interest in emerging and challenging research on automation and machine learning topics. In particular, motion segmentation is a key stage in the design of dynamic data analysis systems. Despite several studies have addressed this issue, there still does not exist a final solution highly compatible with subsequent clustering/classification tasks. In this work, we propose a motion segmentation compatible with kernel spectral clustering (KSC), here termed KSC-MS, which is based on multiple kernel learning and variable ranking approaches. Proposed KSC-MS is able to automatically segment movements within a dynamic framework while providing robustness to noisy environments.
Año de publicación:
2017
Keywords:
- Variable ranking
- Time-varying data
- Motion segmentation
- Kernel spectral clustering
Fuente:
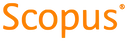
Tipo de documento:
Conference Object
Estado:
Acceso restringido
Áreas de conocimiento:
- Visión por computadora
- Ciencias de la computación
- Simulación por computadora
Áreas temáticas de Dewey:
- Métodos informáticos especiales
- Economía financiera
- Física aplicada

Objetivos de Desarrollo Sostenible:
- ODS 9: Industria, innovación e infraestructura
- ODS 17: Alianzas para lograr los objetivos
- ODS 8: Trabajo decente y crecimiento económico
