Automatic sleep stages classification using EEG entropy features and unsupervised pattern analysis techniques
Abstract:
Sleep is a growing area of research interest in medicine and neuroscience. Actually, one major concern is to find a correlation between several physiologic variables and sleep stages. There is a scientific agreement on the characteristics of the five stages of human sleep, based on EEG analysis. Nevertheless, manual stage classification is still the most widely used approach. This work proposes a new automatic sleep classification method based on unsupervised feature classification algorithms recently developed, and on EEG entropy measures. This scheme extracts entropy metrics from EEG records to obtain a feature vector. Then, these features are optimized in terms of relevance using the Q-α algorithm. Finally, the resulting set of features is entered into a clustering procedure to obtain a final segmentation of the sleep stages. The proposed method reached up to an average of 80% correctly classified stages for each patient separately while keeping the computational cost low.
Año de publicación:
2014
Keywords:
- Relevance analysis
- Signal entropy
- Feature Extraction
- Sleep stages
- feature selection
- Q-α
- Clustering
Fuente:
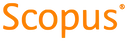
Tipo de documento:
Article
Estado:
Acceso abierto
Áreas de conocimiento:
- Aprendizaje automático
- Ciencias de la computación
Áreas temáticas de Dewey:
- Farmacología y terapéutica
- Fisiología humana
- Métodos informáticos especiales

Objetivos de Desarrollo Sostenible:
- ODS 3: Salud y bienestar
- ODS 12: Producción y consumo responsables
- ODS 9: Industria, innovación e infraestructura
