BLER performance evaluation of an enhanced channel autoencoder
Abstract:
The concept of using autoencoders (AEs) to represent wireless communication systems as an end-to-end reconstruction task that optimizes the transmitter and receiver components simultaneously in a single process has attracted the attention of wireless practitioners worldwide. This is attributable to the flexibility, and convenience of representing complex channel models. However, owing to the characteristics of deep neural networks (DNNs), as the AE learns the representation of the channel, overfitting limits its performance. In this paper, we propose RegAE, a regularized DNN architecture that overcomes the overfitting limitation in AEs and reduces their training complexity, which are characteristics of models with higher dimensions. We demonstrate that RegAE improves the block error rate (BLER) as compared with equivalent models from the literature. Thereby, it achieves a performance (1) better than that of a 4∕7 rate Hamming code with a 16 phase-shift keying (16PSK) modulation under an additive white Gaussian noise (AWGN) channel, (2) comparable to that of a 4∕7 rate maximum likelihood decoding (MLD) with a Eb∕N0 range from 1 dB to 5 dB, and (3) equivalent to that of an uncoded binary phase-shift keying (BPSK) modulation over a Eb∕N0 range from 0 dB to 10 dB.
Año de publicación:
2021
Keywords:
- modulation
- deep learning
- neural network
- Wireless communications
- Autoencoder
- End-to-end learning
Fuente:
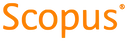
Tipo de documento:
Article
Estado:
Acceso restringido
Áreas de conocimiento:
- Aprendizaje automático
- Algoritmo
Áreas temáticas:
- Ciencias de la computación
- Medios documentales, educativos, informativos; periodismo
- Física aplicada