A new way of finding better neighbors in recommendation systems based on collaborative filtering
Abstract:
One of the biggest problems of the internet is information overload. A way to handle this is Collaborative Filtering. However, it can present problems as they work with large rating matrices, and they are always really sparse. In this paper, we purpose a model that finds the closest neighbors efficiently incorporating dimensionality reduction, using Truncated Singular Value Decomposition which helps with sparse data and avoids noise caused by lack of ratings, then using clustering as we have a dense reduced matrix, and finally applying the correct similarity metric to improve pbkp_redictions. To evaluate the pbkp_rediction quality we use the mean absolute error. The experiments are executed with MovieLens 1M Open Data Set. And to explain the model we use a running example, named datatoy.
Año de publicación:
2019
Keywords:
Fuente:
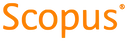
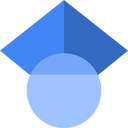
Tipo de documento:
Conference Object
Estado:
Acceso restringido
Áreas de conocimiento:
- Aprendizaje automático
- Ciencias de la computación
- Ciencias de la computación
Áreas temáticas:
- Funcionamiento de bibliotecas y archivos