A nonparametric bootstrap method for spatial data
Abstract:
The aim is to provide a nonparametric bootstrap method for spatial data, which can be either stationary or depart from the stationarity condition due to the presence of a non-constant trend. The proposed technique has been designed to reproduce the variability of the underlying process in an appropriate way, since it takes into account the bias effect due to the use of residuals. In certain cases, other approaches are applicable to spatial data, such as the block bootstrap, although they may fail, for instance, to provide an adequate inference on the variogram. There are also semiparametric resampling methods, based on factoring a parametric estimate of the covariance matrix. However, the results derived from them may be affected by a misspecification of the selected parametric model, as well as by the bias effect induced by the residuals on the estimation. The new procedure has been developed to overcome the aforementioned drawbacks, thus enabling its application to typical inference problems in geostatistics, such as those involving the trend or the second-order structure. The different bootstrap mechanisms have been compared in numerical studies designed to check their performance for a specific problem, namely, the approximation of the bias and the variance of two variogram estimators. In addition, a practical application of the new approach for inference on the variogram of precipitation data has also been included.
Año de publicación:
2019
Keywords:
- Resampling method
- Kernel estimation
- Variogram
- Bandwidth matrix
Fuente:
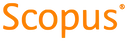
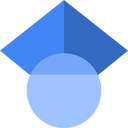
Tipo de documento:
Article
Estado:
Acceso restringido
Áreas de conocimiento:
- Inferencia estadística
Áreas temáticas:
- Métodos informáticos especiales