Benchmarking of Classification Algorithms for Psychological Diagnosis
Abstract:
Generating a clinical diagnosis of a mental disorder is a complex process due to the variety of biological factors that affect this type of condition, so it is necessary that a professional performs a deep evaluation in order to identify and determine the type of disorder that affects the patient. This paper proposes the implementation and comparison of five machine learning algorithms (ML) to generate automatic diagnoses of mental disorders, through the set of symptoms present in a patient. The algorithms selected for comparison are: Support Vector Machine, Logistic Regression, Random Forest, Bayesian Networks, k-Nearest Neighbors (k-NN). The evaluation metrics used on the benchmarked were precision, accuracy, recall, error rate and also we analyzed the ROC curves and the AUC values. The general results show that the Logistic Regression algorithm obtained a better performance with 70.82% of accuracy. The Support Vector Machine model, on the other hand, showed a low performance reaching only 42.99% accuracy.
Año de publicación:
2020
Keywords:
- recall
- ROC
- random forest
- logistic regression
- Precision
- Bayesian networks
- performance
- K-NEAREST NEIGHBORS
- accuracy
- Support Vector Machine
Fuente:
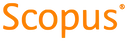
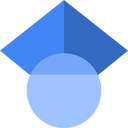
Tipo de documento:
Conference Object
Estado:
Acceso restringido
Áreas de conocimiento:
- Psicometría
- Aprendizaje automático
- Ciencias de la computación
Áreas temáticas:
- Psicología diferencial y del desarrollo
- Psicología
- Enfermedades