Benefits of empirical orthogonal functions in pattern recognition applied to vulnerability assessment
Abstract:
One of the main challenges of power system vulnerability assessment is to handle adequate tools capable of analyzing huge volumes of data in real time. For instance, phasor measurement units (PMUs) offer lots of dynamic time series data regarding phasor electric signals. This dynamic data can be analyzed via signal processing tools, such as Fourier Transform, in order to obtain patterns that alert about possible system stress. However, due to the specific dynamic behavior of post-contingency electric signals, they do not exactly correspond to pure periodic signals. Thus, applying classical Fourier-related transforms might not allow obtaining relevant patterns from these types of signals. In this connection, the present paper proposes to use a well-proven time series data mining technique, called empirical orthogonal functions (EOFs), instead of Fourier-related transforms. EOFs better adapt to the peculiar shape of the post-contingency system variables, which allows getting a better pattern recognition, and so a better vulnerability assessment. With the aim of showing the benefits of EOF, a fair comparison of this data mining tool with Discrete Fourier Transform (DFT) is presented in this paper via three different signal examples.
Año de publicación:
2014
Keywords:
- Electric power systems
- vulnerability assessment
- empirical orthogonal functions (EOF)
- Data Mining
- Discrete Fourier Transform (DFT)
Fuente:
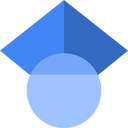
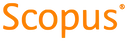
Tipo de documento:
Conference Object
Estado:
Acceso restringido
Áreas de conocimiento:
- Aprendizaje automático
- Optimización matemática
Áreas temáticas:
- Sistemas
- Ciencias sociales
- Enfermedades