A novel framework for improving multi-population algorithms for dynamic optimization problems: A scheduling approach
Abstract:
This paper presents a novel framework for improving the performance of multi-population algorithms in solving dynamic optimization problems (DOPs). The fundamental idea of the proposed framework is to incorporate the concept of scheduling into multi-population methods with the aim to allocate more function evaluations to the best performing sub-populations. Two methods are developed based on the proposed framework, each of which uses a different approach for scheduling the sub-populations. The first method combines the quality of sub-populations and the degree of diversity among them into a single feedback parameter for detecting the best performing sub-population. The second method uses the learning automata as the central unit for performing the scheduling operation. In order to validate the applicability of the proposed methods, they are incorporated into three well-known algorithms for DOPs. The experimental results show the efficiency of the scheduling approach for improving the multi-population methods on the moving peaks benchmark (MPB) and generalized dynamic benchmark generator.
Año de publicación:
2019
Keywords:
- Moving peaks benchmark
- Learning automata
- Evolutionary computation
- Differential Evolution
- Dynamic optimization problems
- scheduling
Fuente:
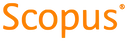
Tipo de documento:
Article
Estado:
Acceso restringido
Áreas de conocimiento:
- Algoritmo
- Algoritmo
- Algoritmo
Áreas temáticas:
- Ciencias de la computación