Beyond model interpretability using LDA and decision trees for α-amylase and α-glucosidase inhibitor classification studies
Abstract:
In this report are used two data sets involving the main antidiabetic enzyme targets α-amylase and α-glucosidase. The pbkp_rediction of α-amylase and α-glucosidase inhibitory activity as antidiabetic is carried out using LDA and classification trees (CT). A large data set of 640 compounds for α-amylase and 1546 compounds in the case of α-glucosidase are selected to develop the tree model. In the case of CT-J48 have the better classification model performances for both targets with values above 80%–90% for the training and pbkp_rediction sets, correspondingly. The best model shows an accuracy higher than 95% for training set; the model was also validated using 10-fold cross-validation procedure and through a test set achieving accuracy values of 85.32% and 86.80%, correspondingly. Additionally, the obtained model is compared with other approaches previously published in the international literature showing better results. Finally, we can say that the present results provided a double-target approach for increasing the estimation of antidiabetic chemicals identification aimed by double-way workflow in virtual screening pipelines.
Año de publicación:
2019
Keywords:
- QSAR
- Decision Trees
- antidiabetic agents
- linear discriminant analysis
Fuente:
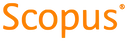
Tipo de documento:
Article
Estado:
Acceso restringido
Áreas de conocimiento:
- Bioquímica
- Aprendizaje automático
Áreas temáticas:
- Programación informática, programas, datos, seguridad
- Artes gráficas y artes decorativas
- Biología