A novel imputation method for missing values in air pollutant time series data
Abstract:
Missing data is a widespread problem that studies in air quality have to deal with. The causes are varied, including sensor malfunctions and errors, power outages, computer system crashes, pollutant levels lower than detection limits, among others. Existing methods of data cleaning focus more on anomaly detection paying less attention to repairing them. Anomaly detection is a common approach used for filtering out dirty data. However, this approach could still result in unreliable data due to the incomplete data resulting from this process. This article presents an approach for repairing continuous sections of missing values in time series of air pollutant data. This study considers two regularized methods, Lasso and Ridge regression, to determine the number of data points forward and backward needed to estimate the value of a missing data point. Lasso presents superiority in front of Ridge regression to generate models for data imputation. The performance of the method was evaluated using the correlation coefficient (R2), the index agreement (d), the mean absolute error (MAE), and root mean square error (RMSE). The proposed imputation method exhibited good results in terms of accuracy and precision in gaps of different lengths. This study proved that the proposed imputation method is useful to impute accurately missing values in air pollution contaminant datasets, and it has the potential to be applied to any dataset configured as a time series.
Año de publicación:
2019
Keywords:
- TIME SERIES
- Imputation method
- Missing values
Fuente:
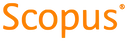
Tipo de documento:
Conference Object
Estado:
Acceso restringido
Áreas de conocimiento:
- Análisis de datos
- Estadísticas
- Ciencia ambiental
Áreas temáticas:
- Ciencias de la computación