A novel method for objective selection of information sources using multi-kernel SVM and local scaling
Abstract:
Advancement on computer and sensing technologies has generated exponential growth in the data available for the development of systems that support decision-making in fields such as health, entertainment, manufacturing, among others. This fact has made that the fusion of data from multiple and heterogeneous sources became one of the most promising research fields in machine learning. However, in real-world applications, to reduce the number of sources while maintaining optimal system performance is an important task due to the availability of data and implementation costs related to processing, implementation, and development times. In this work, a novel method for the objective selection of relevant information sources in a multimodality system is proposed. This approach takes advantage of the ability of multiple kernel learning (MKL) and the support vector machines (SVM) classifier to perform an optimal fusion of data by assigning weights according to their discriminative value in the classification task; when a kernel is designed for representing each data source, these weights can be used as a measure of their relevance. Moreover, three algorithms for tuning the Gaussian kernel bandwidth in the classifier pbkp_rediction stage are introduced to reduce the computational cost of searching for an optimal solution; these algorithms are an adaptation of a common technique in unsupervised learning named local scaling. Two real application tasks were used to evaluate the proposed method: the selection of electrodes for a classification task in Brain–Computer Interface (BCI) systems and the selection of relevant Magnetic Resonance Imaging (MRI) sequences for detection of breast cancer. The obtained results show that the proposed method allows the selection of a small number of information sources.
Año de publicación:
2020
Keywords:
- SUPPORT VECTOR MACHINES
- Multimodality
- Machine learning
- multiple kernel learning
- Source selection
Fuente:
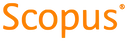
Tipo de documento:
Article
Estado:
Acceso abierto
Áreas de conocimiento:
- Aprendizaje automático
- Algoritmo
Áreas temáticas:
- Programación informática, programas, datos, seguridad
- Biblioteconomía y Documentación informatica
- Funcionamiento de bibliotecas y archivos