A novel statistical based feature extraction approach for the inner-class feature estimation using linear regression
Abstract:
Nowadays, statistical based feature extraction approaches are commonly used in the knowledge discovery field with Machine Learning. These features are accurate and give relevant information of the samples; however, these approaches consider some assumptions, such as the membership of the signals or samples to specific statistical distributions. In this work, we propose to model statistical computation through linear regression models; these models will be divided by classes, in order to increase the inner-class identification likelihood. In general, an ensemble of linear regression models will estimate a targeted statistical feature. In an online deployment, the pool of LR models of a given targeted statistical feature will be evaluated to find the most similar value to the current input, which will be as the estimated of the feature. The proposal is tested with a real world application in traffic network classification. In this case study, fast classification response has to be provided, and statistical based features are widely used for this aim. In this sense, the statistical features must give early signs of the status of the network in order to achieve some objectives such as improve the quality of service or detect malicious traffic.
Año de publicación:
2018
Keywords:
Fuente:
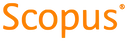
Tipo de documento:
Conference Object
Estado:
Acceso restringido
Áreas de conocimiento:
- Optimización matemática
- Optimización matemática
- Estadísticas
Áreas temáticas:
- Programación informática, programas, datos, seguridad