A pattern-recognition approach for lead-selection in heartbeat detection
Abstract:
In this work, we developed and evaluated an algorithm for selecting the most suitable lead for performing heart-beat detection in ECG signals. For the development and evaluation we used a public dataset of 927 multilead (2-12 leads) stress-test recordings, with manually reviewed heartbeat locations. The algorithm consists of a pattern-recognition block based on features calculated from the RR interval sequence, and a mixture of Gaussian classifier. This block estimates whether the heartbeat is correctly detected, ommited or incorrectly detected. With these estimations, a detection quality index is calculated from the sensitivity (S) and positive pbkp_redictive value (P+) of each lead. With this quality index a decision is made to choose the best lead. Results show that the correct lead has been selected in 70% of the recordings, and in 93% of the recordings the best lead was among the top 3 leads with higher detection quality index. Finally, the selection of the lead with higher quality index produces a gross median S of 100%, with percentile 5 at 99.6, and a gross median P+ of 98.9%, with percentile 5 at 89.2. The algorithm was developed and evaluated using ECG signals, but could be used with other cardiovascular signals as well, being suitable for automatically selecting the best lead/signal, or sorting them for further analysis or manual correction.
Año de publicación:
2014
Keywords:
Fuente:
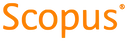
Tipo de documento:
Conference Object
Estado:
Acceso restringido
Áreas de conocimiento:
- Aprendizaje automático
- Ciencias de la computación
Áreas temáticas:
- Métodos informáticos especiales
- Derecho
- Física aplicada