Breast Mass Regions Preprocessing and Classification from Mammograms using Convolutional Neural Networks
Abstract:
Background: Digital mammography imaging is a common diagnostic tool for early detection and classification of early-stage breast lesions. However, noise and artifacts degrade low resolution image. The main goal of this paper is to improve breast image quality resolution which would result in increasing the segmentation and classification accuracy using convolutional neural networks. Method: in this methodology we have used three different breast databases for (1) Manual extraction of regions of interest (RoIs). 2) RoIs data augmentation. 3) RoIs super resolution using EDSR and SR-RDN deep learning algorithms. 4) RoIs segmentation with UNet and SegNet encoder-decoder algorithms, and 5) RoIs classification with ResNet-50. Statistical metrics were used to evaluate the super resolution quality (PSNR and SSIM), segmentation (IoU and Dice) and classification algorithms performance (accuracy, specificity, sensivity). Results: The EDSR (PSNR= 39.05 dB/SSIM= 0.90) method outperformed the visual quality of the new synthetic image with respect to SR-RDN (PSNR= 32.68 dB/SSIM= 0.82). Likewise, UNet outperformed Segnet with an accuracy rate of 0.698, achieving a 75% of classification using ResNet-50. Conclusion: our results showed the EDSR and Unet methods are more robust in getting higher precision in breast RoIs classification.
Año de publicación:
Keywords:
Fuente:
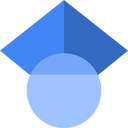
Tipo de documento:
Other
Estado:
Acceso abierto
Áreas de conocimiento:
- Red neuronal artificial
- Ciencias de la computación
Áreas temáticas:
- Métodos informáticos especiales