Bridging the knowledge gap in ridehailing service provision with human-driven fleets: A data mining approach
Abstract:
Ridehailing has undeniably become an important mobility alternative for trip-makers around the globe. Its advent has given rise to a broad range of challenges in policymaking, planning, modelling. Conventional models lack the functionality and structure required to handle complex mobility services such as ridehailing. This complexity resides in service provision processes that involve interdependent features such as matching, rebalancing, dynamic pricing, driver activity. The latter is perhaps the most critical in that the vast majority of providers currently rely on human drivers, hence have very limited control over service fleets. Despite the importance of modelling driver activity, there is a marked dearth of research in this matter, which can in part be attributed to the hype of autonomous vehicles, in part to the lack of data due to stringent data protection policies of (often private) ridehailing providers. In this context, this paper reports data mining efforts to exploit the information available for a conventional ridehailing trip-based dataset from RideAustin. Namely, the paper contributes to the literature with procedures to synthesize unobserved vehicle locations, generate very detailed full-day driver activity logs, identify rebalancing trips from driver logs. These unique contributions provide valuable data that allow a wide range of applications. Relevant examples involve modelling matching and rebalancing mechanisms, or drivers' choice-making situations such as entering active/inactive periods.
Año de publicación:
2020
Keywords:
- Ridehailing
- ridesourcing
- Data Mining
- activity
- Human-driven
- Driver behaviour
Fuente:
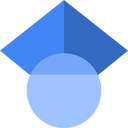
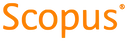
Tipo de documento:
Conference Object
Estado:
Acceso abierto
Áreas de conocimiento:
- Minería de datos
- Ciencias de la computación
Áreas temáticas:
- Sistemas