Building neural-network-based models using nodal and time-series analysis for short-term production forecasting
Abstract:
Implementing asset-wide intelligent digital oilfield (iDOF) solutions, aiming to optimize oil and gas production system in an "intelligent8 manner, requires integrating concepts from different disciplines, such as artificial intelligence. Neural network- (NN-) based models are a form of artificial intelligence, which is a branch of computer science that generates mathematical models that can be "trained" to determine relationships between inputs and outputs, recognize patterns, and perform reliable short-term pbkp_redictions. NN models using real-time data are proven tools for short-term well production forecasting with acceptable accuracy. An oilfield is a hostile environment for even the most robust instrumentation. As a result, technical outages or anomalies can result in lost or poor quality data. Experience shows that many samples in a real-time database are frozen, missing, corrupted, or incorrect. This fact represents the biggest challenge to creating a reliable NN model. However, the models can be trained to correct or estimate missing real-time data. This paper presents a case study where nodal analysis was used to populate missing data used to train NN model, thus improving the reliability of the model. Because nodal analysis is not suitable for pbkp_rediction, time-series analysis was used to assess the impact of historical events, and operational conditions were used to forecast trends. The NN trained with nodal analysis can cover a wide variability spectrum and, when trained with a time-lapse series, can pbkp_redict short-term (30-day) production scenarios by changing highly correlated parameters, such as tubing head pressure (THP) or frequency (Freq). This paper describes training NNs using nodal analysis and time-series analysis to pbkp_redict short-term water cut (WC or BS&W) and liquid flow rate. This technique was applied in over 20 wells with electronic submersible pumps (ESPs) and gas lifts (GLs). The NNs made robust estimates of production rate and an acceptable pbkp_rediction trend for 30 days, even when confronted with flow meter instrumentation failure, lost signals, and out-of-calibration instruments. Hence, the NN served as a "virtual meter," providing instantaneous and accurate estimation of production data.
Año de publicación:
2013
Keywords:
Fuente:
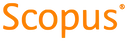
Tipo de documento:
Conference Object
Estado:
Acceso restringido
Áreas de conocimiento:
- Algoritmo
- Aprendizaje automático
Áreas temáticas:
- Ciencias de la computación