Bundle branch blocks classification via ECG using MLP neural networks
Abstract:
This paper proposes a two-stage system based on neural network models to classify bundle branch blocks via electrocardiogram (ECG) analysis. Two artificial neural network (ANN) models have been developed in order to discriminate bundle branch blocks and hemiblocks from normal ECG and other heart diseases. This method includes pre-processing and classification modules. ECG segmentation and wavelet transform were used as pre-processing stage to improve classical multilayer perceptron (MLP) network. A new set of about 800 ECG were collected from different clinics in order to create a new ECG database to train ANN models. For bundle branch blocks classifier in the test phases, the best specificity of all models was found to be 94.56% and the best sensitivity was found to be 92.45%. In the case of hemiblocks classifier, the best results were a sensitivity of 93.26% and a specificity of 92.55%.
Año de publicación:
2014
Keywords:
- Bundle branch blocks
- eCG
- classification
- MLP
- DWT
- Hemiblocks
Fuente:
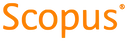
Tipo de documento:
Conference Object
Estado:
Acceso restringido
Áreas de conocimiento:
- Aprendizaje automático
- Ciencias de la computación
Áreas temáticas:
- Ciencias de la computación