A probabilistic logic approach to outcome pbkp_rediction in team games using historical data and domain knowledge
Abstract:
Relational data is structured and, in the real world, ambiguous. Logic can handle relations and probability can handle uncertainty. A probabilistic logic approach to learning can handle both relational structure and uncertainty in the data. Probabilistic logic approach works well with relational data. Incorporating domain knowledge in probabilistic logic approach further enhances learning, improving accuracy. A number of statistical techniques carry out pbkp_redictive analytics based on historical data alone. Soccer, however, is a team game and the outcome of a soccer game depends on how well the team together and the players play against the opponent team. Thus, data about soccer games are better represented in relational form. In the present work, we propose to learn from soccer match data to pbkp_redict their outcomes. We learn a model for the pbkp_rediction of soccer game outcomes, taking into account the history of the matches played by the teams. We frame the background knowledge as rules in the logic program to enhance the pbkp_rediction. Compared to the traditional machine learning approaches to soccer game outcome pbkp_rediction, probabilistic logic approach is found to result in significant improvement in pbkp_rediction accuracy.
Año de publicación:
2021
Keywords:
- UNCERTAINTY
- Probabilistic logic
- Parameter learning
- pbkp_rediction
- Soccer match
- MLE
- Historical data
Fuente:
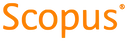
Tipo de documento:
Article
Estado:
Acceso restringido
Áreas de conocimiento:
- Aprendizaje automático
- Ciencias de la computación
- Análisis de datos
Áreas temáticas:
- Sistemas
- Métodos informáticos especiales
- Funcionamiento de bibliotecas y archivos