C-MADA: Unsupervised Cross-Modality Adversarial Domain Adaptation framework for Medical Image Segmentation
Abstract:
Deep learning models have obtained state-of-the-art results for medical image analysis. However, CNNs require a massive amount of labelled data to achieve a high performance. Moreover, many supervised learning approaches assume that the training/source dataset and test/target dataset follow the same probability distribution. Nevertheless, this assumption is hardly satisfied in real-world data and when the models are tested on an unseen domain there is a significant performance degradation. In this work, we present an unsupervised Cross-Modality Adversarial Domain Adaptation (C-MADA) framework for medical image segmentation. C-MADA implements an image-level and feature-level adaptation method in a two-step sequential manner. First, images from the source domain are translated to the target domain through an unpaired image-to-image adversarial translation with cycle-consistency loss. Then, a U-Net network is trained with the mapped source domain images and target domain images in an adversarial manner to learn domain-invariant feature representations and produce segmentations for the target domain. Furthermore, to improve the network’s segmentation performance, information about the shape, texture, and contour of the pbkp_redicted segmentation is included during the adversarial training. C-MADA is tested on the task of brain MRI segmentation from the crossMoDa Grand Challenge and is ranked within the top 15 submissions of the challenge.
Año de publicación:
2022
Keywords:
- image segmentation
- Domain Adaptation
- Medical image analysis
- Generative Adversarial Networks
- unsupervised learning
Fuente:
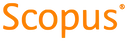
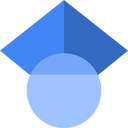
Tipo de documento:
Conference Object
Estado:
Acceso restringido
Áreas de conocimiento:
- Ciencias de la computación
Áreas temáticas:
- Medicina y salud
- Enfermedades
- Ciencias de la computación