COVID-19 ResNet: Residual neural network for COVID-19 classification with three-step Bayesian optimization
Abstract:
COVID-19 is an infectious disease caused by a novel coronavirus called SARS-CoV-2. The first case appeared in December 2019, and until now it still represents a significant challenge to many countries in the world. Accurately detecting positive COVID-19 patients is a crucial step to reduce the spread of the disease, which is characterized by a strong transmission capacity. In this work we implement a Residual Convolutional Neural Network (ResNet) for an automated COVID-19 diagnosis. The implemented ResNet can classify a patient's Chest-Xray image (CXR) as COVID-19 positive, pneumonia caused from another virus or bacteria, or healthy. Moreover, to increase the accuracy of the model and overcome the data scarcity of COVID-19 images, a personalized data augmentation strategy using a three-step Bayesian hyperparameter optimization approach is applied to enrich the dataset during the training process. The proposed COVID-19 ResNet achieves a 94% accuracy, 95% recall, and 95% F1-score in the test set. Furthermore, we also provide insight into which data augmentation operations are successful in increasing CNN performance when doing medical image classification with COVID-19 CXR.
Año de publicación:
2020
Keywords:
Fuente:
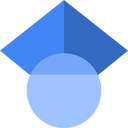
Tipo de documento:
Other
Estado:
Acceso abierto
Áreas de conocimiento:
- Aprendizaje automático
- Algoritmo
Áreas temáticas:
- Métodos informáticos especiales
- Ciencias de la computación
- Funcionamiento de bibliotecas y archivos