Capacity and retrieval of a modular set of diluted attractor networks with respect to the global number of neurons
Abstract:
The modularity and hierarchical structures in associative networks can replicate parallel pattern retrieval and multitasking abilities found in complex neural systems. These properties can be exhibited in an ensemble of diluted Attractor Neural Networks for pattern retrieval. It has been shown in a previous work that this modular structure increases the single attractor storage capacity using a divide-and-conquer approach of subnetwork diluted modules. Each diluted module in the ensemble learns disjoint subsets of unbiased binary patterns. The present article deals with an ensemble of diluted Attractor Neural Networks which is studied for different values of the global number of network units, and their performance is compared with a single fully connected network keeping the same cost (total number of connections). The ensemble system more than doubles the maximal capacity of the single network with the same wiring cost. The presented approach can be useful for engineering applications to limited memory systems such as embedded systems or smartphones.
Año de publicación:
2017
Keywords:
- Storage capacity
- Ensembled learning
- Diluted connectivity
- Divide-and-conquer approach
- Network size
- Hopfield network
Fuente:
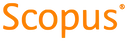
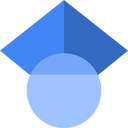
Tipo de documento:
Article
Estado:
Acceso restringido
Áreas de conocimiento:
- Aprendizaje automático
Áreas temáticas:
- Ciencias de la computación