A score-driven model of short-term demand forecasting for retail distribution centers
Abstract:
Forecasting is one of the fundamental inputs to support planning decisions in retail chains. Frequently, forecasting systems in retail are based on Gaussian models, which may be highly unrealistic when considering daily retail data. In addition, the majority of these systems rely on point forecasts, limiting their practical use in retailing decisions, which often requires the full pbkp_redictive density for decision making. The main contribution of this paper is the modeling of daily distribution centers (DCs) level aggregate demand forecasting using a recently proposed framework for non-Gaussian time series called score-driven models or Generalized Autoregressive Score (GAS) models. An experimental study was carried out using real data from a large retail chain in Brazil. A log-normal GAS model is compared to usual benchmarks, namely neural networks, linear regression, and exponential smoothing. The results show the GAS model is a competitive alternative to retail demand forecasting in daily frequency, with the advantage of producing a closed form pbkp_redictive density by construction.
Año de publicación:
2021
Keywords:
- Log-normal pbkp_redictive density
- Distribution center aggregate data
- Retail demand forecasting
- Score-driven models
Fuente:
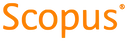
Tipo de documento:
Article
Estado:
Acceso restringido
Áreas de conocimiento:
- Optimización matemática
Áreas temáticas:
- Dirección general