A semantic approach to improve neighborhood formation in collaborative recommender systems
Abstract:
Automatic recommenders are now omnipresent in e-commerce websites, as selecting and offering to users products they may be interested in can considerably increase sales revenue. The most popular recommendation strategy is currently considered to be the collaborative filtering technique, based on offering to the user who will receive the recommendation items that were appealing to other individuals with similar preferences (the so-called neighbors). On the other hand, its principal obstacle is the sparsity problem, related to the difficulty to find overlappings in ratings when there are many items. As the product catalogue of these sites gets more and more diverse, a new problem has arisen that happens when users share likings for lots of products (for which they are reckoned to be neighbors) but they differ in products similar to the one that is being considered for recommendation. They are fake neighbors. Narrowing the range of products on which similarities between users are sought can help to avoid this, but it usually causes a worsening of the sparsity problem because the chances of finding overlappings gets lower. In this paper, a new strategy is proposed based on semantic reasoning that aims to improve the neighborhood formation in order to overcome the aforementioned fake neighborhood problem. Our approach is aimed at making more flexible the search for semantic similarities between different products, and thus not require users to rate the same products in order to be compared. © 2014 Elsevier Ltd. All rights reserved.
Año de publicación:
2014
Keywords:
- Semantic similarity metric
- Fake neighborhood
- semantic reasoning
- Sparsity problem
- COLLABORATIVE FILTERING
Fuente:
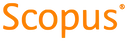
Tipo de documento:
Article
Estado:
Acceso restringido
Áreas de conocimiento:
- Inteligencia artificial
- Ciencias de la computación
Áreas temáticas:
- Funcionamiento de bibliotecas y archivos
- Métodos informáticos especiales