A spatio-temporal Bayesian model for syndromic surveillance: properties and model performance
Abstract:
Methods In this paper, we propose an inference model for determining the location of outbreaks of epidemics in a network of nodes. In our setting, the network is the NC counties where the basic model incorporates spatial geographical relationships between the counties. The model is epidemiological, by choice, to process daily flu counts from the counties in order to infer when an outbreak of flu is present in a county that is distinguishable from background counts. The methodology incorporates Gaussian Markov random field (GMRF) and spatio-temporal conditional autoregressive (CAR) modeling. Results The methodology has some nice features including timely detection of outbreaks, robust inference to model misspecification, reasonable pbkp_rediction performance as well as attractive analytical and visualization tool to assist public health authorities in risk assessment. Based on extensive simulation studies and synthetic data generated from a dynamic SIR model, we demonstrated that the model is capable of capturing outbreaks rapidly, while still limiting false positives.
Año de publicación:
Keywords:
Fuente:
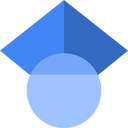
Tipo de documento:
Other
Estado:
Acceso abierto
Áreas de conocimiento:
Áreas temáticas:
- Programación informática, programas, datos, seguridad
- Otros problemas y servicios sociales
- Probabilidades y matemática aplicada