A strategy based on local similarity enhancement for computational segmentation of the superior vena cava in cardiac computed tomography
Abstract:
he article proposes a strategy to segment the superior vena cava (VCS) into 20-dimen- sion (3-D) images of multi-layer computed tomography, corresponding to the complete cardiac cycle of a patient. This strategy consists of the stages of pre-processing, segmentation and intonation of parameters. The pre-processing stage is divided into two phases. In the first phase, called the filtering phase, a technique called local similarity enhancement (LSE) is used in order to reduce the impact of the artifacts and to attenuate noise in the quality of the images. This technique combines an averaging filter, an edge detector filter (called black top hat) and a Gaussian filter (GF). In the second, identified as a region of interest (ROI) definition phase, we consider filtered images, least squares vector support machines and a priori information to isolate the anatomical structures that surround the VCS. On the other hand, during the 3-D segmentation stage a clustering algorithm, called region growth (RG), is implemented, which is applied to the preprocessed images. During the intonation of parameters, of the proposed strategy, the Dice coefficient (Dc) is used to compare the segmentations of the superior vena cava, obtained automatically, with the segmentation of the VCS generated manually by a cardiologist. The combination of parameters that generated the highest Dc considering the instant of diastole is then applied to the remaining 19 three-dimensional images, obtaining an average Dc higher than 0.88 which indicates a good correlation between the segmentations generated by the expert cardiologist and those produced by the strategy developed.
Año de publicación:
2017
Keywords:
- Tomography
- segmentation
- Local similarity Enhancement
- Superior vena cava
Fuente:
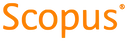
Tipo de documento:
Article
Estado:
Acceso restringido
Áreas de conocimiento:
Áreas temáticas:
- Fisiología humana
- Medicina y salud
- Ciencias de la computación