Cardiac Fibrosis Detection Applying Machine Learning Techniques to Standard 12-Lead ECG
Abstract:
Hypertrophic cardiomyopathy (HCM) is a myocardial disorder that affects 0.2% of the population and it is genetically transmitted. Several ECG findings have been related to the presence of fibrosis in other cardiac diseases, but data for HCM in this setting are lacking. Our hypothesis is that fibrosis affects the electrical cardiac propagation in patients with HCM in a relatively specific way and that this effect may be detected with suitable postprocessing applied to the ECG signals. We used 43 standard 12-lead ECGs from patients with previous clinical diagnosis of HCM. Principal Component Analysis (PCA) was applied by combining the ECG-leads oriented to different anatomic regions, hence assessing the potential fibrosis effects in the resulting leads for postprocessing convenience. Linear classifier of Support Vector Machine type were used with several statistics extracted from the resulting PCA-components, including normalized power, standard deviation, kurtosis, skewness, and local maxima. Results reached 75.0% sensitivity, 80.0% specificity, 85.7% positive pbkp_redictive value, 66.7% negative pbkp_redictive value, and 76.9% accuracy in our database. There is evidence that myocardial fibrosis can be detected in patients with HCM by postprocessing their ECG signals.
Año de publicación:
2018
Keywords:
Fuente:
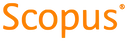
Tipo de documento:
Conference Object
Estado:
Acceso abierto
Áreas de conocimiento:
- Enfermedad cardiovascular
- Aprendizaje automático
Áreas temáticas de Dewey:
- Enfermedades
- Medicina y salud
- Ciencias de la computación