Cellular estimation Gaussian algorithm for continuous domain
Abstract:
Optimization algorithms are important in problems of pattern recognition and artificial intelligence, i.e., the image recognition, face recognition, data analysis, optical recognition, etc. Estimation distribution algorithms (EDAs) is kind of optimization algorithms based on substituting the crossover and mutation operators of the Genetic Algorithms by the estimation and later sampling the probability distribution learned from the selected individuals. However, a weakness of these algorithms is the efficiency in terms of the number of evaluations of the fitness function. In this paper, a Cellular Gaussian Estimation Algorithm (CEGA) for solving continuous optimization problems is proposed. CEGA is derived from evidence-based learning of independence and decentralized schemes of local populations. The experimental results showed that the present proposal reduces the number of evaluations of the fitness function in the search for optimums, maintaining its effectiveness in comparison to other algorithms of state-of-Art using the same benchmark of continuous functions.
Año de publicación:
2019
Keywords:
- Probabilistic graph model
- Learning
- GAUSSIAN networks
- Cellular EDA
Fuente:
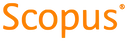
Tipo de documento:
Article
Estado:
Acceso restringido
Áreas de conocimiento:
- Algoritmo
- Ciencias de la computación
- Algoritmo
Áreas temáticas:
- Ciencias de la computación