Characterization and classification of intracardiac atrial fibrillation signals using the time-singularity multifractal spectrum distribution
Abstract:
The analysis of intracardiac signals, or electrograms (EGM), is one of the most promising tools to guide catheter ablation of atrial fibrillation and improve the success of this procedure. Given the nonlinear nature of EGM signals, several studies have conducted fractal and multifractal analyses to extract nonlinear features related with critical activity. However, the fractal exponent or the multifractal spectrum fail to provide information about the temporal behavior of these signals. To overcome this limitation, the Time-Singularity Multifractal Spectrum Distribution (TS-MFSD) was recently introduced. In this paper, a feature extraction scheme is proposed to compute descriptors from the TS-MFSD that could be used in a classification scheme. The results show that features extracted from the TS-MFSD would serve to classify EGM signals into four classes depending on their level of fragmentation. In addition, the k-Nearest Neighbors and Support Vector Machines classifiers employed in this study, along with the optimal feature subset, achieved an accuracy of 81.37±0.95% and 83.35±1.04%, respectively. This finding is comparable with those of other works that have used features based on the morphology of local activation waves and amplitude thresholds.
Año de publicación:
2021
Keywords:
- Nonlinear signal processing
- Time-singularity multifractal spectrum distribution
- Multifractal spectrum
- Atrial Fibrillation
Fuente:
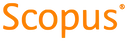
Tipo de documento:
Article
Estado:
Acceso restringido
Áreas de conocimiento:
- Enfermedad cardiovascular
- Procesamiento de señales
Áreas temáticas:
- Fisiología humana
- Enfermedades