A voice analysis approach for recognizing Parkinson's disease patterns
Abstract:
Many of the patients diagnosed with Parkinson's disease (PD) do not know they have it until the most severe symptoms appear, sometimes they must wait months or even years to get the correct diagnosis, so detection in its early stage is important to improve the quality of life of patients and families. We propose the creation of a model based on supervised learning, to learn the patterns associated with the voice of PD patients. We used 1400 voice recordings of PD patients and controls which were preprocessed, further were obtained 70 features for each recording, and then we used a supervised learning algorithms such as a Multilayer Perceptron (MLP), Random Forest (RF), Logistic Regression (LR), and Support Vector Machines (SVM) to classify the data between patients and controls. From all machine learning models evaluated the SVM model showed the best performance, with an accuracy of 88%. This work presents the possibility to incorporate the voice analysis as digital biomarker to facilitate diagnosis in PD.
Año de publicación:
2021
Keywords:
- Machine learning
- PÁRKINSON
- Voice Analysis
Fuente:
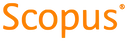
Tipo de documento:
Conference Object
Estado:
Acceso abierto
Áreas de conocimiento:
- Aprendizaje automático
Áreas temáticas de Dewey:
- Enfermedades