Classification models applied to uncertain data
Abstract:
In the field of learning models, the quality directly depends on the training data. That is the reason why data preparation is one of the stages in the knowledge extraction process where more time is invested. In fact, the most common scenario consists in a training created under perfect conditions. However, the situation is often entirely different during the model deployment phase, since, in the real world, data usually contain noise, there may be missing or incorrect values, or even be uncertain, in the sense that we do not know their exact value, but have an approximate knowledge of its value. In this paper, we will study how to apply the learning models to uncertain data. Specifically, we will focus on classification problems in which uncertainty is only present in numerical attributes and present a new approach to apply classification learned models. Experimental results show that the accuracy achieved by our methods improve the case of having maximum uncertainty. Random Forest has a 3.60% control of uncertainty when its maximum value is achieved. Also, there is a higher level of degradation of 5.59% and 9.60% for both Decision Trees and Naive Bayes.
Año de publicación:
2019
Keywords:
- Classification Models
- random forest
- learning models
- Decision Trees
- Naïve Bayes
Fuente:
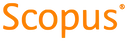
Tipo de documento:
Conference Object
Estado:
Acceso restringido
Áreas de conocimiento:
- Aprendizaje automático
- Inferencia estadística
Áreas temáticas de Dewey:
- Programación informática, programas, datos, seguridad
- Métodos informáticos especiales
- Ciencias sociales

Objetivos de Desarrollo Sostenible:
- ODS 9: Industria, innovación e infraestructura
- ODS 17: Alianzas para lograr los objetivos
- ODS 4: Educación de calidad
