Classification of metabolic syndrome subjects and marathon runners with the k-means algorithm using heart rate variability features
Abstract:
In this paper, we have applied the k-means clustering algorithm to classify three study groups (people with metabolic syndrome, marathon runners, and sedentary subjects) that underwent a 5-sample 2-hour oral glucose tolerance test (OGTT). For this purpose, time-domain, frequency-domain and non-linear parameters of the heart rate variability (HRV), extracted from ECG recordings acquired at five different instants of the OGTT, were used as unidimensional observations to the k-means algorithm. Specifically, standard deviation of RR intervals (SDNN), root-mean-square differences of successive RR intervals (RMSSD), frequency power in the low frequency (LF) and high-frequency (HF) bands, LF/HF ratio, Poincaré descriptors SD1 and SD2, fractal scaling exponents α1 and α2, and approximate entropy were used as observations. Experiments were carried out with k = 2 and k = 3 clusters and using the squared Euclidean and Cityblock distances. Results showed that the Cityblock distance outperformed the squared Euclidean distance for this kind of observations. In addition, the parameter SDNN at the end of the OGTT gave the best classification performance (69.2%). Parameters SDNN, RMSSD, SD1 and SD2 at fast and at 30 min of the test differentiated subjects with metabolic syndrome with classification a performance greater than 60%.
Año de publicación:
2016
Keywords:
Fuente:
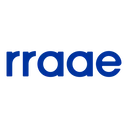
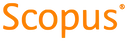
Tipo de documento:
Conference Object
Estado:
Acceso restringido
Áreas de conocimiento:
- Fisiología
- Aprendizaje automático
- Nutrición
Áreas temáticas:
- Farmacología y terapéutica
- Fisiología humana
- Ciencias de la computación