Classification techniques in breast cancer diagnosis: A systematic literature review
Abstract:
Data mining (DM) consists in analysing a set of observations to find unsuspected relationships and then summarising the data in new ways that are both understandable and useful. It has become widely used in various medical fields including breast cancer (BC), which is the most common cancer and the leading cause of death among women worldwide. BC diagnosis is a challenging medical task and many studies have attempted to apply classification techniques to it. The objective of the present study is to identify studies on classification techniques in BC diagnosis and to analyse them from three perspectives: classification techniques used, accuracy of the classifiers, and comparison of performance. We performed a systematic literature review (SLR) of 176 selected studies published between January 2000 and November 2018. The results show that, of the nine classification techniques investigated, artificial neural networks, support vector machines and decision trees were the most frequently used. Moreover, artificial neural networks, support vector machines and ensemble classifiers performed better than the other techniques, with median accuracy values of 95%, 95% and 96% respectively. Most of the selected studies (57.4%) used datasets containing different types of images such as mammographic, ultrasound, and microarray images.
Año de publicación:
2021
Keywords:
- Data Mining
- diagnosis
- Breast Cancer
- classification
Fuente:
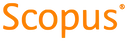
Tipo de documento:
Article
Estado:
Acceso restringido
Áreas de conocimiento:
- Cáncer
- Aprendizaje automático
- Cáncer
Áreas temáticas:
- Enfermedades
- Medicina y salud
- Funcionamiento de bibliotecas y archivos